Are your campaigns effective? Do you have the right Attribution model to find out?
- William Lum
- Jul 18, 2021
- 5 min read
Updated: Jun 24, 2024
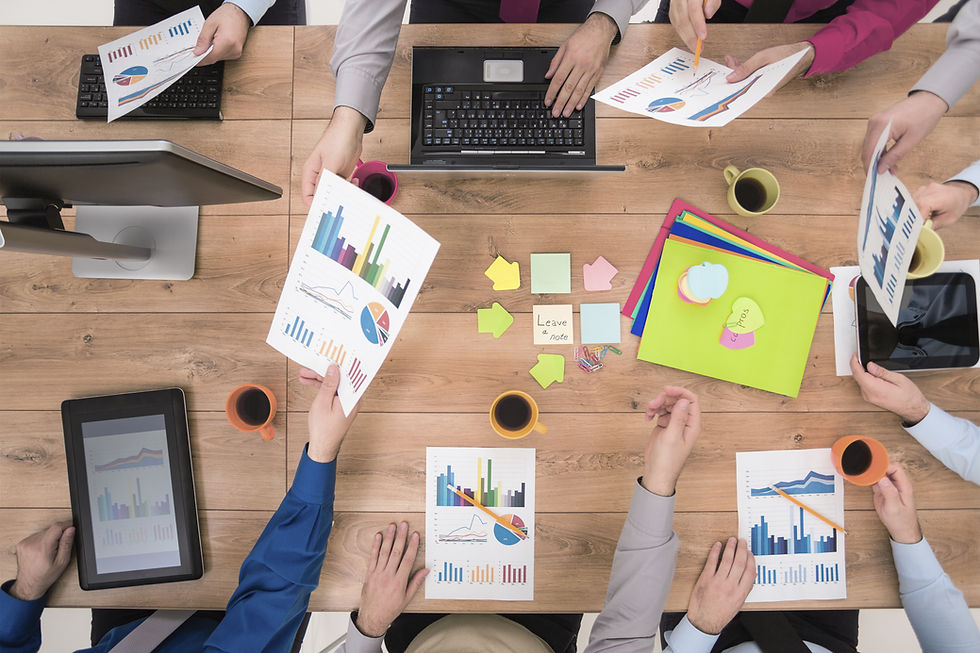
This has been a bit of a hot-button topic for me because many marketers are doing this wrong and don't even realize it. It's partly because this process is inherently complicated and most marketing teams aren't equipped with the analytical skills to validate if the models are working.
What is Marketing Attribution?
Marketing Attribution is the practice of evaluating marketing touchpoints to understand which channels and content (assets) had the greatest impact at moving the prospect to convert. Convert typically means Opportunity won but some models can also be more granular and examine conversion from one key stage to another.
Knowing what channels/assets worked for which stage helps us know where to focus future resources (people, effort, budget, etc). Do more of what works (i.e. create more assets that are similar to the well-received ones) and/or try something new to improve where performance is weak (i.e. look at channels that have underperformed).
Commonly seen Attribution Models
Data science has proven there are more accurate ways to do marketing attribution. Data collection and computational power are no longer a limitation on the types of models we can use. It's surprising many of the Attribution Models still used today are rules-based (poor accuracy but easy to understand). These models build on some well-intentioned logic and assumptions made by the marketer but typically give a skewed view of what it thinks is "working". These have 2 parts to the logic:
tiering of activity (Marketer's assumptions)
prioritization base on deal value
For simplicity, the model may look only at contacts associated with the Deal or it will look at all contacts at the company that had activity within X time frame before the Opportunity creation date.
Let's take a look at some common models. In each chart, we show the Attribution of the campaign responses (A-J) received overtime... Selection of which responses to evaluate are based on the model, the Opportunity Creation Date, and any parameters of the model that define how far back to look.
First Touch

First Touch, as the name suggests picks
the very first response and applies the value of the entire deal to it. The thinking is the first campaign responded to is the most impactful as it is how we gained their info and can continue to communicate with them. However, this means that no other campaigns will be evaluated... reducing the number of campaigns that can hope to see rightful attribution. I have seen some modified versions of First Touch where the model limits how far back it will look for a response (i.e. 30 days before Opportunity creation data). While know what campaign got a contact to respond and enter our database is valuable... using First Touch as an attribution method is not.
Last Touch

Last Touch, similar to First Touch only assigns value to one response for that prospect, the last one just before the Opportunity creation date. The thinking here is, the very last response must be most important as that is the marketing campaign that finally got them to become a customer and thus should get the credit. This was also a very early model used when collecting response data was difficult and doing additional math was a luxury.
Linear
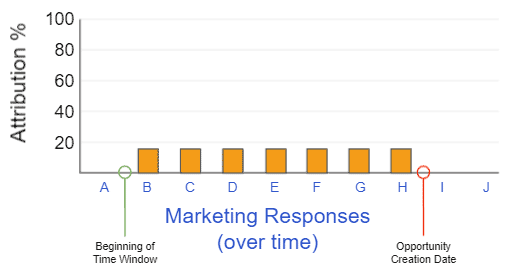
Linear came about when tracking campaign responses became easier. It is straightforward and distributes the value of the deal across all campaign responses equally. This approach assumes all campaign responses are equal and thus the opportunity value is divided equally. Typically there is a time box (i.e. the model will look back 1.5x the avg. sales cycle length) for campaign responses. This model is not a terrible place to start if you can limit the contacts to the people involved on the client-side in the deal. Over many deals, patterns should emerge Assets and Channels (with highest accumulated value).
U-shaped
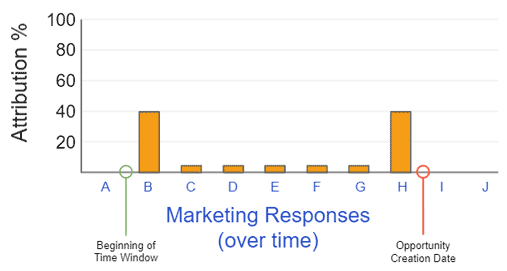
U-shaped is a hybrid of the First Touch, Last Touch, and Linear models. Combining the assumptions that most campaigns should be treated equally, except the First and Last responses are special (for the reasons stated previously in the First Touch and Last Touch model descriptions).
W-shaped
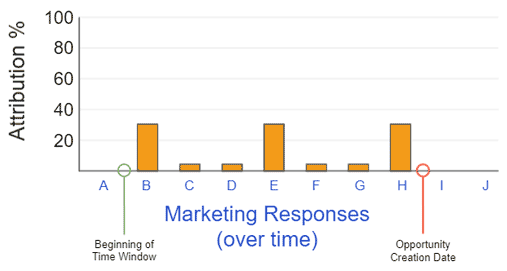
W-shaped was seen as an "enhancement" to the U-shaped model... building on the assumptions, the first and last responses are special and most other responses are not... except for the middle campaign that "sustained" the interest... and thus should be treated special like the first and last responses. Honestly, this starts to feel a little like magical snake oil is being sold to unsuspecting marketers that don't have strong analytical acumen.
Time Decay

Time decay introduced another way to think of responses and was a recognition that we are constantly inundated with marketing and the ones we remember most often are the ones that happened most recently. Time Decay favours more recent responses over older ones.
Categorical

Categorical is one of the strangest and most polarizing models I've seen. Responses are grouped and weighted by type of marketing activity (event, conference, email, ad, etc). These are highly biased to the marketer's opinion. In this example, In-person events (E and G) are deemed to be worth more than Webinars (C) which are deemed to be worth more than Email responses (B, D, F, and H).
All these models try to apply logical reasoning to predict what is important. And you can see the reasoning evolve and become more complex. Some of the more complex rules-based models today use a combination of the above models to try to replicate what the marketing thinks is happening during the buying process with prospects. And that there might be the problem.
Machine Learning models
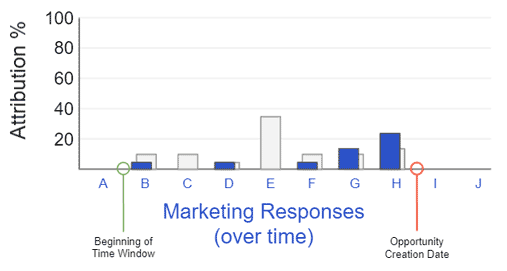
Machine Learning models takes a data science approach. With these models, we don't assume the importance of any of the responses. We use lots of data for wins and losses to model the overall Attribution amount of the campaigns. A simplified explanation of the logic... We examine prospects with very similar lists of responses except for one campaign response. In one group are those with that one additional campaign response and in the other are those without. By looking at the conversion rate in each group, tells us how much of an effect that campaign response had on conversion rate. A common downfall of the rules-based models is the inability to remove responses that appear just as often in lost deals as won deals. Those responses in Predictive models will also be accounted for and should show little or no effect on conversion and attribution.
While rules-based models are very easy to understand and implement, machine learning models are far superior in terms of accuracy. It's like using an abacus in a calculator world.
(Are you still using rules-based Attribution? When will you be migrating?)
Understanding what campaigns are effective is the heavy lifting but we also need to consider cost efficiently. Perhaps that's a topic for another post.
(Comment below if you'd like to discuss further.)
Aside: To make them more understandable there are several interesting Interpretable Machine Learning initiatives underway that have output to help us understand why something is weighted/score that way.
Comentarios