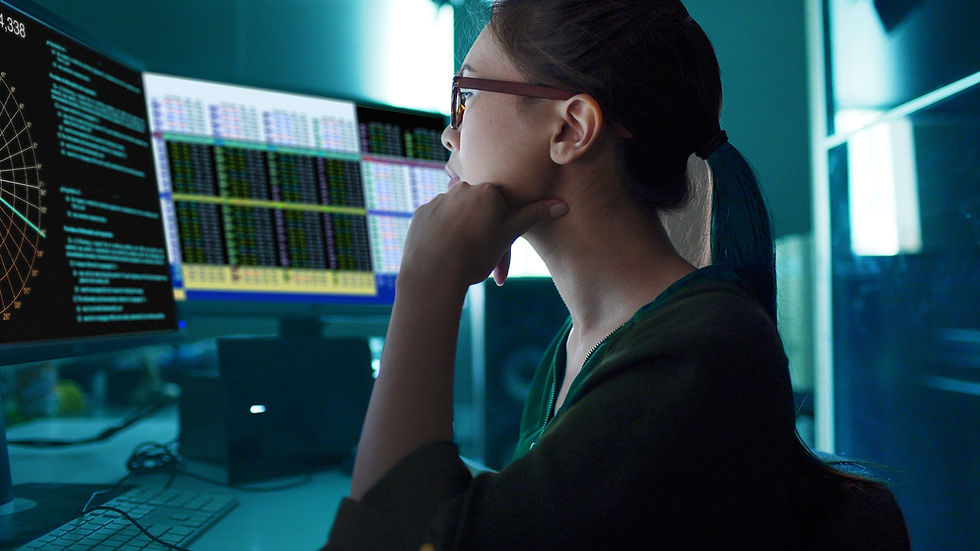
When things are new they are hard to use and require specialized knowledge to operate. Over time, as common user errors are seen, safeguards and usability gets added, and usage becomes democratized.
It wasn't that long ago that website could only be built by hand-coding HTML. The first tools were geared to help programmers to speed up their workflow. Today we have tools for non-technical end-users truly democratizing access to building websites. These users are not burdened by the syntax of the code. Instead, they could instead spend that energy purely on the visitor experience and we have an explosion of creativity all over the web.
"Time to results is key"
We are better off with technology in the hands of the marketing user and we use specialized technical resources to make customizations that need advanced skills. Time to results is key and this model of democratized access enables Citizen Data Scientist, marketing users that learn enough data science to perform basic data science tasks without a deep understanding of the math used vs trying to align team projects to get time with the Data Science team periodically. This way you may not get all of the value but your time to value is measured in days.
"... scarcity of data scientist..."
Another driving factor is the scarcity of data scientists and data engineers. While more data scientists are being trained every year. The need for their skills continues to grow: https://searchbusinessanalytics.techtarget.com/feature/Data-scientist-shortage-leaves-organizations-uncertain
We can't wait for a few to be competitive... we have to spread the work across more enabled people.
"... problems are best solved by the subject matter experts."
There is an added benefit of involving the subject matter experts (SMEs). The constraints (logical and data) are best understood by the subject matter experts... and thus the problems are best solved by the SMEs. Data scientists are experts in data science, not the business and there is an inevitable iterative approach to solving the problem as SMEs look at the analysis and recognize limitations in the data and process.
Few companies have the luxury of having an army of data scientists ready to work on your projects. This framework focuses on getting more people involved and if there are data scientists available leveraging their limited time to solving specialized problems.
Business understanding – What does the business need?
Marketing SMEs and Analysts are essential
Data understanding – What data do we have / need? Is it clean?
Marketing SMEs know the data quality and when it's available and how it's used
Data preparation – How do we organize the data for model?
Training to enable Analysts and technical Marketing SMEs to increase the number of resources able to do Data Engineering (widen bandwidth)
Marketing Automation and integration specialist are great candidates as they have some overlapping skills
some complex prep may still need Data Engineers
Modelling – What modelling techniques should we apply?
Enabling SMEs and Analysts as Citizen Data Scientists will help get a basic model (getting 80% of the value now is better than waiting months or years for 100% of the value
Optimization and refinement of the modelling technique will need the expertise of Data Scientist that the nuances of the math and techniques
Evaluation – Which model best meets the business objectives?
Marketing SMEs and Analysts are essential, enabling SMEs and Analysts as Citizen Data Scientists shortens the iterative cycles
Deployment – How do stakeholders access the results?
Application Specialists, Analysts, Developers understand the systems and processes the model/data needs to connect to, enabling them for this Data Engineering work shortens time frames
Enabling Citizen Data Scientists/Engineers means picking a low code or no-code solution. If you have Developers you might be able to use low-code solutions... but this still means there is still some lost time coordinating between developers and Marketing SMEs. This also means there is a smaller pool of resources you can leverage but the upside is you can take on more technically challenging work. KNIME is focused on helping streamline developer workflow and aligns well with the developer thought process. The benefit of enabling SMEs is now you have a large pool of resource to draw from but you will need a solution that is oriented around a business problem vs a technical feature... this way the interface leads the user through common steps in the solution and has guard rails to keep them from making technical mistakes. Alteryx Designer has an interface that is focused on the data and is oriented around the business problem.
(Comment below if you'd like to see a post about resources to learn data science, hands-on data science tutorials, or other topics)
There are many techniques and methods that can be used to solve problems through data but choosing the ideal one can be confusing for those new to Data Science.
Comments